Highlights:
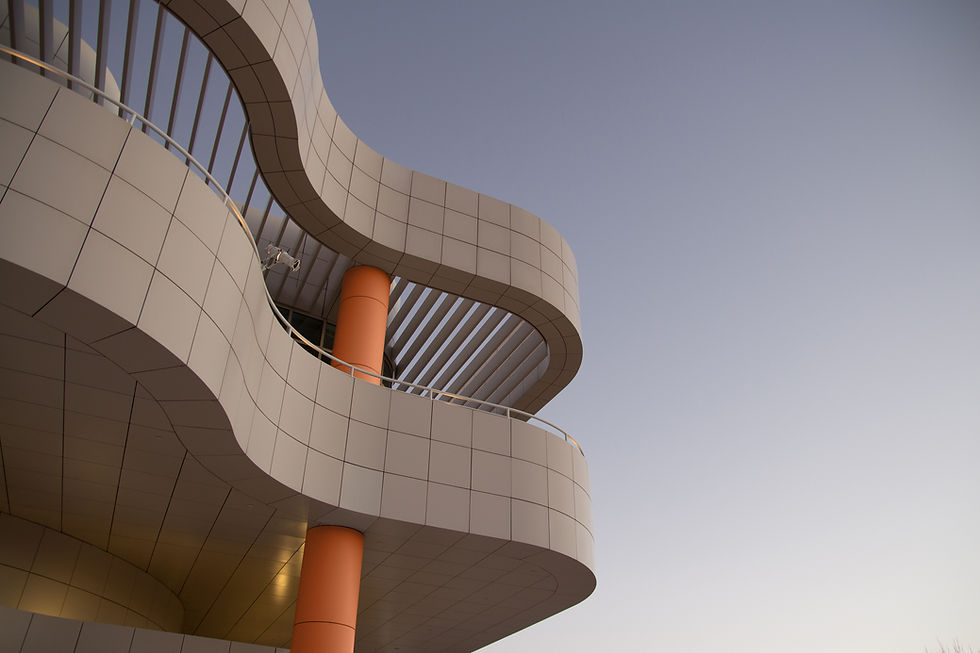
The Rise of AutoML in Data Science Workflows
5 days ago
4 min read
0
2
0
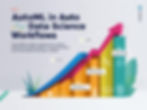
Data Science has become a driving force behind decision-making processes across industries. With the proliferation of data, organizations are increasingly relying on data-driven insights to gain competitive advantages. However, the process of extracting these insights is not trivial. It involves collecting, cleaning, and analyzing vast amounts of data, building predictive models, and interpreting results. Traditionally, this entire workflow required the expertise of highly skilled data scientists. But now, Automated Machine Learning, or AutoML, is revolutionizing this landscape.
What is AutoML?
AutoML refers to the process of automating the end-to-end process of applying machine learning to real-world problems. This includes everything from data preprocessing and feature engineering to model selection, training, tuning, and deployment. AutoML platforms aim to make machine learning more accessible to non-experts and to increase productivity for seasoned data scientists.
AutoML tools reduce the need for manual intervention, saving time and resources. These tools offer the promise of democratizing machine learning, allowing domain experts without a deep background in data science to build and deploy effective models.
Evolution of AutoML
The concept of AutoML has evolved rapidly in the last decade. Initially, it started with simple hyperparameter tuning frameworks like Grid Search and Random Search. As the demand for automated solutions grew, more advanced tools emerged. Google’s AutoML, H2O.ai’s Driverless AI, DataRobot, and Microsoft’s Azure AutoML are now at the forefront of this movement.
These platforms offer drag-and-drop interfaces and support a wide array of machine learning tasks, including classification, regression, clustering, and even time series forecasting. Some also incorporate explainability features, helping users understand how models make decisions.
Key Components of AutoML
AutoML is a combination of multiple automated steps. Let’s break down the components:
1. Data Preprocessing
AutoML tools can automatically handle missing values, outliers, and inconsistent data. They also apply transformations like normalization, encoding categorical variables, and feature scaling.
2. Feature Engineering
Feature engineering is often the most time-consuming part of a data science project. AutoML systems use statistical techniques and domain-specific heuristics to generate new features or select the most relevant ones.
3. Model Selection
AutoML platforms test multiple algorithms on the dataset, including decision trees, random forests, gradient boosting machines, neural networks, and more. They then select the best-performing model based on specific metrics.
4. Hyperparameter Optimization
Advanced AutoML tools use techniques like Bayesian Optimization and Genetic Algorithms to find the best hyperparameters, improving the accuracy and robustness of the model.
5. Model Evaluation
AutoML evaluates models using cross-validation and various performance metrics such as accuracy, precision, recall, and F1-score. This ensures the model generalizes well on unseen data.
6. Model Deployment
Some platforms support seamless deployment of models into production environments, including cloud and on-premises solutions.
Benefits of AutoML
The integration of AutoML into data science workflows offers numerous benefits:
1. Efficiency and Speed
AutoML significantly reduces the time needed to develop machine learning models. Tasks that previously took weeks can now be done in hours.
2. Accessibility
Non-technical users, such as business analysts, can now create and evaluate models, reducing dependence on specialized data science teams.
3. Scalability
AutoML allows companies to scale their data science initiatives without proportionally increasing headcount.
4. Consistency
Automated workflows reduce human error and lead to more consistent results.
5. Cost-Effectiveness
By reducing the time and expertise required, AutoML helps organizations cut costs related to machine learning development.
Challenges and Limitations
Despite its benefits, AutoML is not without challenges:
1. Lack of Flexibility: AutoML tools may not be suitable for highly complex or custom modeling tasks.
2. Interpretability: Some AutoML-generated models, especially deep learning models, act like black boxes, making it hard to explain predictions.
3. Overfitting Risks: Without proper oversight, AutoML tools may overfit to training data.
4. Data Privacy Concerns: Using cloud-based AutoML tools may raise privacy issues, especially when dealing with sensitive data.
5. Loss of Control: Automation may limit the fine-tuning and customization capabilities available to experienced data scientists.
Use Cases of AutoML
1. Retail: Retailers use AutoML to predict customer churn, recommend products, and optimize inventory.
2. Healthcare: AutoML assists in diagnosing diseases, predicting patient outcomes, and personalizing treatment plans.
3. Finance: Banks and fintech companies leverage AutoML for credit scoring, fraud detection, and algorithmic trading.
4. Marketing: AutoML helps marketers personalize campaigns, segment customers, and forecast ROI.
5. Manufacturing: Predictive maintenance and quality assurance are areas where AutoML shows a significant impact.
The Role of AutoML in the Future of Data Science
As data continues to grow in volume and complexity, the demand for scalable and efficient machine learning solutions will only increase. AutoML is poised to play a central role in meeting this demand. It will not replace data scientists but will augment their capabilities, allowing them to focus on higher-level strategic tasks and complex problems that require human intuition.
Moreover, AutoML will contribute to the democratization of AI, enabling small and medium-sized enterprises to leverage the power of machine learning without massive investments in talent and infrastructure.
Educational and Career Implications
With the rise of AutoML, educational institutions and training programs are also evolving. Data science training programs now include AutoML as a core module, teaching students how to use these tools effectively.
If you're looking to build a career in this ever-evolving field, enrolling in a comprehensive data science training course in Noida, Delhi, Lucknow, Meerut, and more cities in India can be a strategic first step. These programs are designed to provide hands-on experience with modern tools, including AutoML platforms.
Conclusion
The rise of AutoML marks a significant milestone in the evolution of data science. By automating complex processes, it empowers a broader range of users to extract insights from data efficiently and accurately. While AutoML is not a one-size-fits-all solution and cannot entirely replace the expertise of skilled data scientists, it serves as a powerful complement to human ingenuity.
As businesses strive to become more data-driven, the adoption of AutoML will only grow. Understanding its capabilities, limitations, and applications is essential for anyone involved in the data science ecosystem. Whether you're a beginner or a seasoned professional, embracing AutoML is no longer optional—it is a necessary step towards staying relevant in the data-driven future.