Highlights:
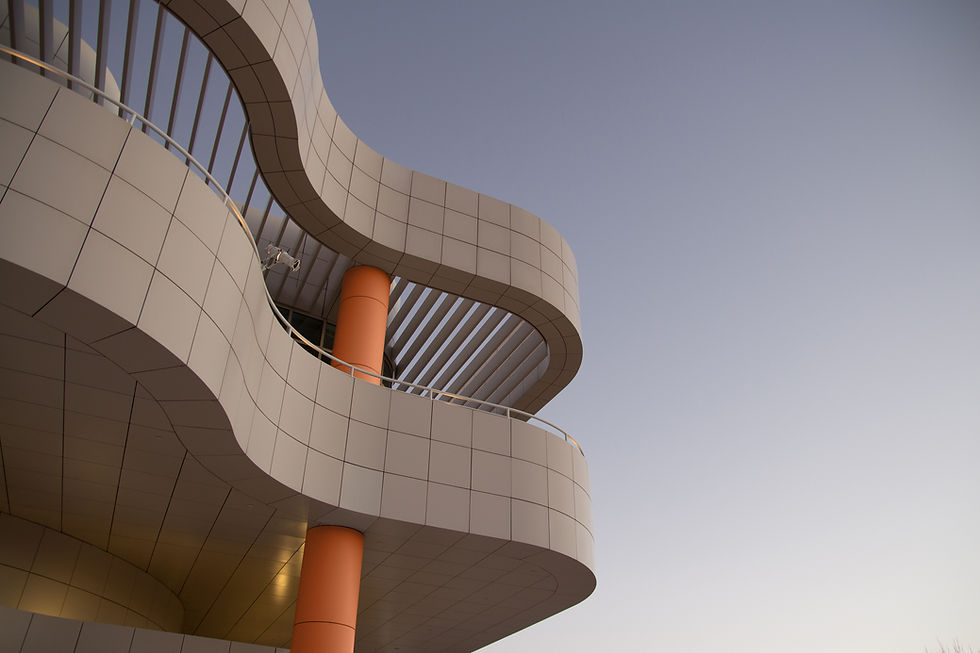
Data Science in Banking: Trends, Benefits, and Roadblocks to Overcome
Nov 26, 2024
4 min read
0
4
0
In the banking sector, institutions are turning to data science to drive innovation, improve customer experiences, and streamline operations. By leveraging vast amounts of financial data, banks can make smarter decisions, enhance risk management, and offer more personalized services. However, the integration of data science into banking isn't without its challenges. In this article, we'll explore the latest trends in data science within the banking sector, the benefits it offers, and the roadblocks that need to be overcome for successful implementation.
Key Trends in Data Science in Banking
Artificial Intelligence (AI) and Machine Learning (ML) AI and machine learning are increasingly becoming integral to data science in banking. These technologies are used to analyze customer behavior, detect fraudulent activities, and automate tasks such as customer service (through chatbots) and risk assessment. Machine learning algorithms also help banks identify patterns and trends that were previously undetectable, leading to more accurate forecasts and better decision-making.
Predictive Analytics for Customer Insights Banks are utilizing predictive analytics to anticipate customer needs and provide tailored financial products. By analyzing transaction history, spending behavior, and other financial data, predictive models help banks offer personalized services such as custom loan offers, credit card promotions, and investment advice. This not only enhances customer satisfaction but also improves customer retention.
Fraud Detection and Prevention Fraud detection is one of the most critical areas where data science is making a significant impact. Advanced machine learning models can analyze transaction data in real-time, identifying unusual patterns or behaviors that may indicate fraud. These systems can alert banks immediately, preventing potential losses and enhancing the security of banking transactions.
Blockchain and Data Security Blockchain technology, combined with data science, is playing a vital role in improving security and transparency in banking. Banks are exploring blockchain for secure data storage, real-time transactions, and reducing fraud. By leveraging the distributed ledger system, financial institutions can ensure that sensitive customer data remains secure while maintaining transparency.
Robotic Process Automation (RPA) RPA is increasingly used in banks to automate repetitive tasks such as data entry, account reconciliation, and transaction processing. By using data science to streamline these processes, banks can improve efficiency, reduce human error, and allow employees to focus on higher-value tasks like customer service and decision-making.
Benefits of Data Science in Banking
Improved Customer Experience With data science, banks can offer a more personalized experience by analyzing customer data to deliver targeted products and services. Personalized financial advice, custom loan options, and tailored investment strategies can help banks build stronger relationships with their clients.
Enhanced Risk Management By using predictive analytics, banks can better assess risk and make more informed decisions. Data science models can analyze market trends, historical data, and customer behavior to predict potential financial risks, allowing banks to act proactively and mitigate losses.
Operational Efficiency Data science enables banks to streamline their operations by automating manual tasks, identifying inefficiencies, and improving resource allocation. This leads to reduced operational costs and improved productivity, benefiting both the bank and its customers.
Fraud Prevention and Security As mentioned earlier, data science plays a crucial role in fraud detection. Advanced algorithms can identify fraudulent patterns in real-time, allowing banks to take immediate action. This enhances security and builds customer trust in the institution’s ability to safeguard their financial information.
Better Decision Making With access to vast amounts of data, banks can make better, data-driven decisions. Whether it’s setting interest rates, determining loan eligibility, or forecasting market trends, data science ensures that decisions are based on insights derived from accurate and up-to-date information.
Roadblocks to Overcome
Data Privacy and Security Concerns One of the primary challenges facing data science in banking is the management of customer data privacy and security. With sensitive financial information being collected, stored, and analyzed, banks must ensure they comply with data protection regulations such as GDPR. Any security breach could result in significant financial losses and damage to the institution's reputation.
Integration with Legacy Systems Many banks still operate on legacy systems that were not built to handle the vast amounts of data required for advanced analytics. Integrating new data science tools and technologies with these outdated systems can be complex and costly. Banks need to modernize their infrastructure to fully harness the potential of data science.
Lack of Skilled Talent Data science requires specialized skills, and there is currently a shortage of qualified professionals in the field. Banks may face difficulty hiring or training employees who have expertise in machine learning, AI, and data analytics. This talent gap could slow down the adoption of data science technologies in the banking sector.
Regulatory Challenges The banking industry is highly regulated, and any new technology or system must comply with these regulations. Banks must navigate complex legal frameworks and ensure that their data science initiatives align with regulatory requirements, such as those related to data usage, fraud prevention, and customer protection.
Data Quality Issues The effectiveness of data science models relies on the quality of the data being used. Banks may encounter issues with inaccurate, incomplete, or outdated data that can hinder the performance of analytics systems. Ensuring data integrity is crucial for deriving reliable insights.
Conclusion
Data science is transforming the banking sector by enabling institutions to make smarter decisions, improve customer experiences, and enhance operational efficiency. However, the journey towards fully harnessing the power of data science is not without its challenges. Banks must address issues such as data privacy, legacy system integration, and talent shortages to fully leverage the potential of data science. As the demand for skilled professionals grows, enrolling in a Data Science Training Institute in Nagpur, Noida, Lucknow, Meerut, Mumbai and more cities in India can help bridge the talent gap by providing individuals with the necessary expertise. By overcoming these roadblocks, banks can pave the way for a more secure, efficient, and customer-centric future in the financial industry.