Highlights:
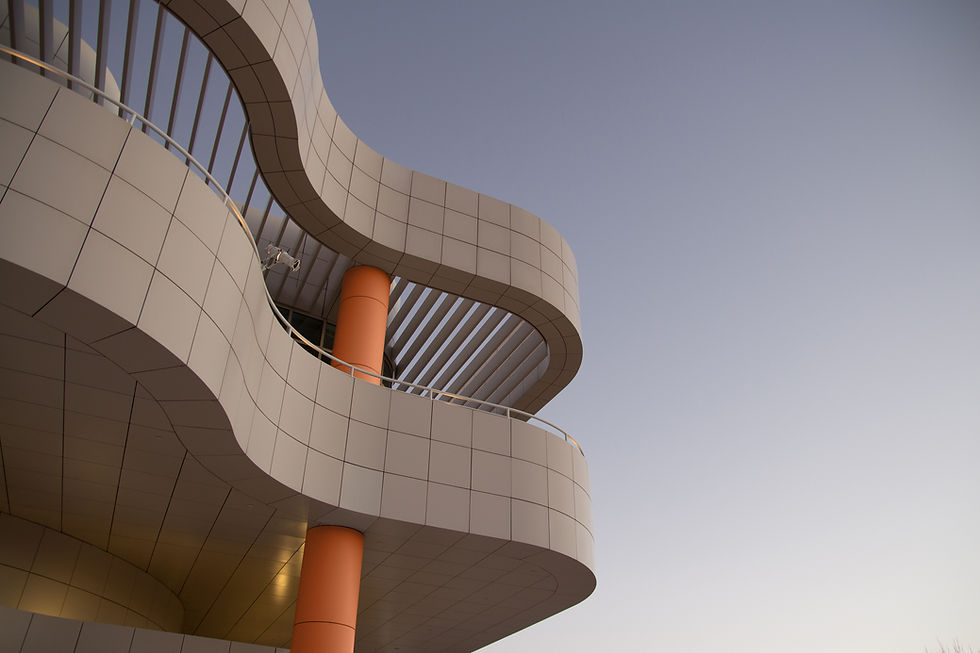
Ethics in Data Science and Proper Privacy and Usage of Data
Sep 19, 2024
4 min read
0
3
0
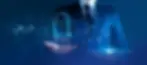
Data Science has emerged as one of the most powerful and transformative fields in the modern era, driving innovation and enabling businesses, governments, and individuals to make data-driven decisions. However, with great power comes great responsibility. The massive amounts of data generated, collected, and analyzed raise significant ethical concerns, especially regarding privacy, security, and the proper use of data. As data science continues to evolve, the need for ethical guidelines and responsible data usage becomes increasingly critical.
Ethics in Data Science
Ethics in data science refers to the moral principles that guide how data is collected, processed, analyzed, and applied. These principles are essential for ensuring that data is used responsibly and in a way that does not harm individuals, communities, or society at large.
The core ethical concerns in data science revolve around transparency, fairness, accountability, and privacy. In order to uphold these values, data scientists must ensure that their work is free from bias, that the data they handle is treated with care, and that they communicate their findings honestly and clearly.
Key Ethical Issues in Data Science
Bias and Fairness: One of the most pressing ethical concerns in data science is bias. Data is often collected from specific sources or populations, and if not carefully managed, it can lead to biased outcomes. For example, an AI system trained on biased data can make unfair or discriminatory decisions, particularly in sensitive areas like hiring, lending, or criminal justice.
To ensure fairness, data scientists must take steps to detect and mitigate bias, both in the data and in the algorithms they develop. This involves using diverse data sets, testing for bias, and being transparent about potential limitations in their models.
Transparency and Accountability: Data scientists must be transparent about the methods they use and the potential impacts of their work. This means clearly explaining how algorithms and models are developed, how data is processed, and what assumptions are made. Being transparent allows stakeholders to understand and trust the results of data analysis.
Accountability also plays a crucial role. Data scientists must take responsibility for the outcomes of their models and the decisions based on their analyses. If a model produces harmful results, it is the responsibility of the data scientist to identify the problem and take corrective action.
Informed Consent and Privacy: Privacy is one of the most significant ethical concerns in data science. As organizations collect vast amounts of personal data, they must ensure that individuals are aware of how their data is being used and have given their informed consent. This includes making privacy policies clear, allowing individuals to opt out of data collection, and safeguarding sensitive information from misuse.
Informed consent is not just about getting permission—it’s about ensuring that individuals understand the potential risks and implications of sharing their data. Without proper consent, data collection practices can become invasive and violate individuals' rights to privacy.
The Importance of Data Privacy
Data privacy involves protecting the personal and sensitive information of individuals. In the age of big data, privacy concerns are growing, as more personal data is being stored, shared, and analyzed. From social media to healthcare data, the misuse of this information can lead to identity theft, discrimination, and other forms of harm.
Ensuring proper privacy practices requires organizations to implement strong data protection measures, such as encryption, anonymization, and secure data storage. These measures help prevent unauthorized access and ensure that sensitive data is only used for its intended purpose.
Best Practices for Proper Data Usage
To address ethical concerns and ensure the proper use of data, organizations and data scientists should follow best practices, including:
Data Minimization: Collect only the data that is necessary for the analysis. Avoid gathering unnecessary or sensitive information that could lead to privacy violations.
Anonymization and Encryption: Protect individuals’ identities by anonymizing data whenever possible. Encrypt sensitive data to prevent unauthorized access during transmission or storage.
Transparent Policies: Clearly communicate how data will be used, and obtain informed consent from individuals before collecting or analyzing their data. Ensure privacy policies are easily accessible and understandable.
Regular Audits and Monitoring: Conduct regular audits of data usage and analysis practices to identify any potential ethical issues, such as bias or privacy risks. Continuous monitoring helps maintain accountability.
Ethical Frameworks and Guidelines: Adopt industry-standard ethical frameworks, such as the Data Science Code of Ethics or guidelines from organizations like the IEEE or ACM. These frameworks provide a foundation for ethical decision-making in data science.
The Role of Regulations
Governments and regulatory bodies have introduced policies to protect individuals’ privacy and ensure the ethical use of data. Regulations like the General Data Protection Regulation (GDPR) in Europe and the California Consumer Privacy Act (CCPA) in the U.S. set strict guidelines on how personal data should be collected, stored, and processed. These regulations provide individuals with more control over their data, requiring organizations to be transparent and accountable for their data practices.
Compliance with these regulations is not just a legal requirement; it is also an ethical imperative for data scientists and organizations to prioritize data privacy and ensure that data is handled responsibly.
Conclusion
Ethics in data science is not just a theoretical concern but a practical necessity. As data becomes increasingly central to decision-making processes in various sectors, ensuring the responsible use of data is essential to protect individuals’ rights and prevent harm. Data scientists, organizations, and policymakers must work together to address ethical challenges, prioritize privacy, and establish transparent, fair, and accountable data practices. For aspiring data professionals, enrolling in the Best Data Science Training Course in Delhi, Noida, Lucknow, Meerut and more cities in India can provide both the technical skills and ethical understanding required to navigate these challenges effectively. Only by doing so can we unlock the full potential of data science while safeguarding the well-being of society.
4o