Highlights:
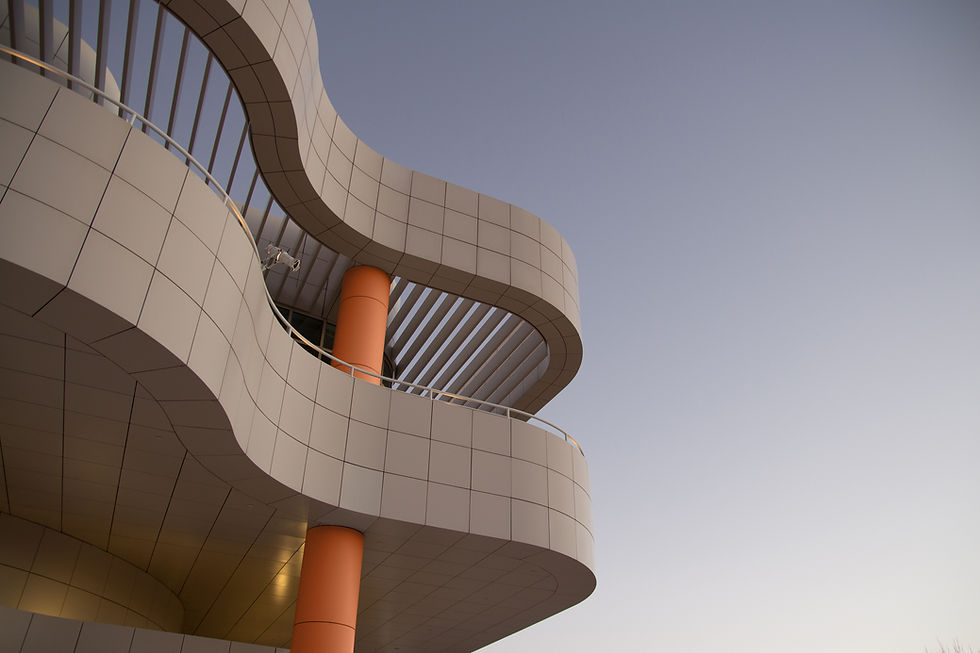
Ethics of Data Analytics: Balancing Technology and Morality
6 hours ago
4 min read
0
2
0

Introduction
Data has become the most valuable asset for organizations worldwide in today's digital era. With the rapid advancement of technologies like Artificial Intelligence (AI), Machine Learning (ML), and Big Data, businesses can now collect, process, and analyze massive amounts of data to drive smarter decisions and optimize operations. However, this surge in data usage raises a significant ethical dilemma: how do we balance the immense power of data analytics with the moral responsibilities that come with it?
The ethics of data analytics is not merely a theoretical discussion but a pressing real-world concern. Issues surrounding privacy, consent, bias, transparency, and accountability have become increasingly prominent. As data analytics becomes deeply integrated into sectors like healthcare, finance, law enforcement, and education, the stakes are higher than ever.
Data Analytics Means and Its Ethical Landscape
Data analytics involves examining large datasets to uncover hidden patterns, correlations, and insights that can inform decision-making. It enables predictive modeling, customer behavior analysis, fraud detection, and more. But behind every dataset lies personal, sensitive, or proprietary information, which brings about ethical considerations.
The ethical landscape of data analytics includes:
Data Privacy: Respecting individuals' rights over their personal information.
Informed Consent: Ensuring individuals are aware of and agree to how their data will be used.
Bias and Fairness: Preventing discriminatory outcomes from skewed datasets.
Transparency: Making data processes and intentions clear to stakeholders.
Accountability: Holding data handlers responsible for misuse or breaches.
Privacy: The Cornerstone of Ethical Data Use
One of the most significant concerns in data analytics is privacy. With the collection of personal information from websites, apps, IoT devices, and social media, individuals often unknowingly provide access to sensitive data.
Key ethical issues include:
Surveillance and Intrusion: Over-collection of data can lead to a surveillance society.
Lack of Anonymity: Even anonymized data can sometimes be re-identified.
Unauthorized Sharing: Selling or sharing user data without consent.
Case Example: The Facebook-Cambridge Analytica scandal highlighted how unethical data use can influence political outcomes and breach public trust.
Organizations must adopt stringent privacy policies, adhere to data protection regulations like GDPR, and ensure minimal and necessary data collection.
Informed Consent and Transparency
Informed consent implies that users are made fully aware of how their data will be used, and they willingly agree to it. Unfortunately, this is rarely the case in real life.
Problems include:
Complicated Privacy Policies: Often lengthy and filled with jargon.
Implicit Consent: Users unknowingly permit by simply using a service.
Ethical data analytics must simplify consent mechanisms and use clear, user-friendly language. Transparency should extend to disclosing algorithms used, the rationale behind decisions, and potential consequences.
Bias in Data and Algorithms
Data is not neutral. It reflects historical and societal biases. When this biased data feeds into algorithms, it can lead to discriminatory results.
Examples include:
Hiring Algorithms: Favoring certain genders or ethnic groups.
Predictive Policing: Targeting specific communities unfairly.
Bias can be unintentional, arising from incomplete data or flawed assumptions. Ethical practices demand regular audits, diverse datasets, and inclusive design teams to mitigate bias.
Accountability in the Age of Algorithms
As machines make more decisions, the question arises: Who is responsible when things go wrong?
Accountability challenges:
Opaque Algorithms: Black-box models that are hard to interpret.
Distributed Responsibility: When multiple stakeholders are involved.
Ethical frameworks require companies to ensure explainability and take full responsibility for the outcomes of their analytics systems. This also includes redress mechanisms for those affected by algorithmic decisions.
Ethical Use of Data in Key Sectors
Healthcare:
Ethical concerns include patient confidentiality, informed consent for research data, and potential misuse of genetic data.
Finance:
Fair lending practices, fraud detection without false accusations, and transparency in credit scoring.
Law Enforcement:
Predictive policing tools must be carefully designed to avoid racial profiling.
Education:
Student data must be protected, and analytics should support personalized learning without unfairly labeling or tracking.
Regulatory and Governance Frameworks
To navigate the ethics of data analytics, several frameworks and regulations have been developed:
General Data Protection Regulation (GDPR): Emphasizes consent, right to access, and data minimization.
California Consumer Privacy Act (CCPA): Grants rights to know, delete, and opt out.
OECD Privacy Guidelines: An Early international standard for data handling.
However, laws often lag behind technological innovation. Organizations must go beyond compliance to adopt ethical leadership and governance.
Building an Ethical Data Culture in Organizations
Creating a data-driven yet ethical organization requires a culture shift:
Leadership Commitment: Top management must prioritize ethical considerations.
Ethics Training: Educating teams on biases, privacy, and fairness.
Ethical Review Boards: Internal committees to assess data projects.
Diverse Teams: Bringing varied perspectives to design and implementation.
Embedding ethics into the organizational DNA ensures long-term sustainability and trust.
Ethical Challenges with Emerging Technologies
With the advent of AI, IoT, and biometrics, new ethical dilemmas emerge:
Deepfake Detection and Misuse
Emotion Recognition Technologies
Mass Surveillance through Smart Devices
These technologies push the boundaries of what is possible but also demand stricter moral oversight.
The Role of Data Analysts and Professionals
Ethics is not just a corporate issue; it’s personal. Data professionals must act as stewards of data.
Responsibilities include:
Raising concerns about unethical practices.
Ensuring fairness in data collection and modeling.
Advocating for transparency and inclusivity.
Educational institutions also play a role. A data analytics course institute in Delhi, Noida, Lucknow, Meerut, and more cities in India may include ethics modules to equip future analysts with the tools to navigate complex moral landscapes.
Conclusion
As data analytics continues to revolutionize industries, it is crucial to remember that with great power comes great responsibility. Balancing technology with morality requires conscious efforts from individuals, organizations, governments, and educational institutions alike.
Ethical data practices build trust, foster innovation, and promote inclusivity. By embedding ethical principles into the very foundation of data projects, we not only prevent harm but also unlock the full potential of analytics in a way that benefits society as a whole.