Highlights:
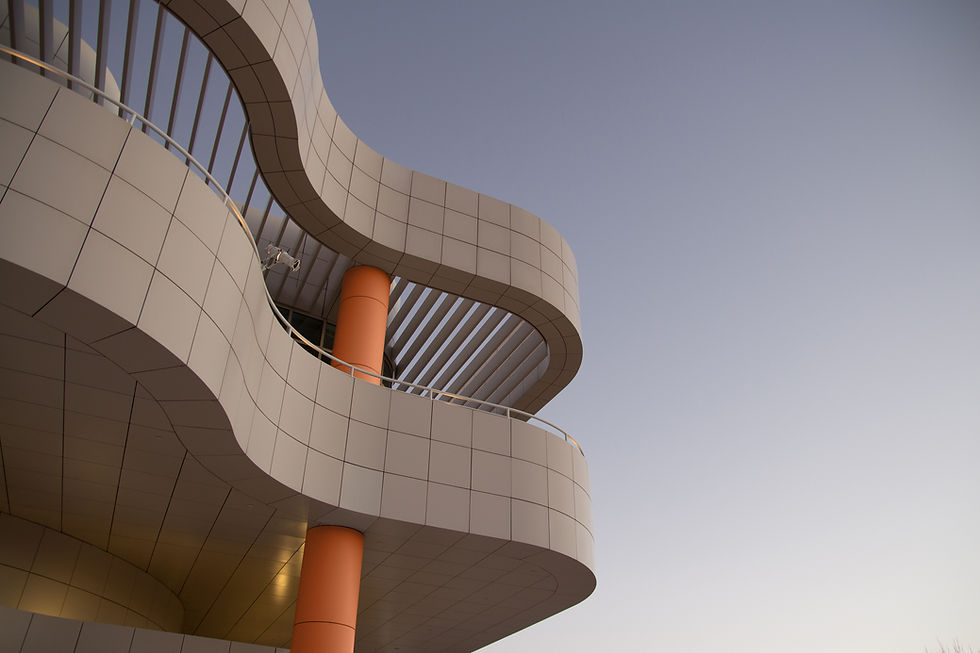
Exploring the Ethical Implications of Data Analytics
Sep 24, 2024
5 min read
0
6
0
The importance of understanding and addressing ethical implications has become paramount in data analytics. As organizations collect, analyze, and interpret massive amounts of data, the responsibility to do so ethically cannot be overlooked. While data analytics provides profound insights into consumer behavior, healthcare trends, financial forecasts, and more, it also brings to light significant challenges that must be confronted. These challenges include issues of privacy, bias, consent, and the potential misuse of data. In this article, we explore the ethical concerns surrounding data analytics, the principles that guide responsible use, and how businesses can maintain transparency while leveraging data for growth.
1. The Role of Ethics in Data Analytics
Ethics in data analytics refers to the moral principles and guidelines that dictate how data should be handled, interpreted, and applied. With data breaches, misuse of personal information, and algorithmic bias making headlines, the role of ethics has never been more critical.
Businesses and organizations must strive to create a culture of ethical data use, where the rights of individuals are respected and protected. The absence of ethical considerations in data practices can result in harm to individuals and communities, legal repercussions, and loss of public trust. Hence, the question arises: how can companies navigate the thin line between innovation and ethical responsibility?
Transparency and Informed Consent
One of the foundational elements of ethical data usage is transparency. When organizations collect data, they must be upfront about what they are collecting, why they are collecting it, and how they plan to use it. Customers should not be left in the dark regarding the extent of data collection. Informed consent is critical—individuals must be given the option to agree (or not) to the collection and usage of their data. This practice not only builds trust but also ensures compliance with data protection regulations such as GDPR and CCPA.
Data Minimization
Ethical data collection practices also emphasize data minimization, a principle that suggests only collecting the data that is strictly necessary for a given purpose. Collecting unnecessary information increases the risk of data leaks, misuse, and unethical exploitation of data. By adhering to the principle of data minimization, organizations reduce these risks while maintaining focus on their primary goals.
The Risks of Bias in Data Analytics
Data analytics, while powerful, is not immune to biases. Bias can enter the process in various ways: through the data itself, the algorithms used to process the data, or the interpretations made by analysts. Ethical concerns about bias are especially pronounced in areas such as healthcare, criminal justice, finance, and hiring processes, where biased analytics can have far-reaching and damaging consequences.
Algorithmic Bias
One of the most concerning forms of bias in data analytics is algorithmic bias, which occurs when algorithms produce skewed results due to faulty assumptions or incomplete data. For instance, if historical data reflects societal inequalities, an algorithm trained on that data is likely to perpetuate those inequalities. Such biases can disproportionately affect vulnerable populations, leading to discriminatory outcomes in areas like credit scoring, hiring decisions, and criminal justice sentencing.
Mitigating Bias
To mitigate the risks of bias, organizations must rigorously test their algorithms, incorporate diverse datasets, and continually audit their analytics processes. It is also crucial to involve interdisciplinary teams—including ethicists, sociologists, and legal experts—in the development and deployment of algorithms. Doing so ensures a well-rounded approach to identifying and correcting biases before they cause harm.
Data Privacy and the Individual
Data privacy is perhaps the most significant ethical issue in the world of data analytics. In a time when personal information is considered a valuable commodity, ensuring the privacy of individuals has become a monumental challenge. Data analytics often involves the collection and analysis of sensitive personal data such as health records, financial transactions, and location history. The misuse or unauthorized sharing of such data can have devastating consequences for individuals, including identity theft, discrimination, and loss of privacy.
Anonymization and Data Masking
To address privacy concerns, companies should adopt techniques such as data anonymization and data masking. These processes involve removing personally identifiable information (PII) from datasets so that individuals cannot be traced or identified. While these methods help protect privacy, they are not foolproof, as advances in technology may allow previously anonymized data to be re-identified.
Balancing Privacy and Innovation
Balancing the need for innovation with respect for privacy is one of the most significant challenges in data analytics. As organizations strive to create more personalized and targeted services, they must ensure that data privacy remains a top priority. Adopting privacy by design—a framework that integrates privacy into the entire data lifecycle—can help organizations navigate this balance effectively.
Ethical Governance and Regulation
Given the ethical complexities associated with data analytics, regulatory frameworks are increasingly being developed to protect individuals and ensure that businesses act responsibly. Legislation such as the General Data Protection Regulation (GDPR) in Europe and the California Consumer Privacy Act (CCPA) in the United States imposes strict guidelines on data collection, storage, and usage. These laws aim to give individuals more control over their personal data and hold organizations accountable for ethical lapses.
Corporate Responsibility and Governance
Organizations must go beyond regulatory compliance and take a proactive approach to ethical governance. This involves establishing internal policies and guidelines that promote responsible data practices, as well as appointing data protection officers (DPOs) or ethics boards to oversee data use. Regular audits, risk assessments, and adherence to ethical frameworks such as the Ethics Guidelines for Trustworthy AI are also essential in maintaining ethical standards.
The Role of Data Ethics in Corporate Social Responsibility (CSR)
Incorporating data ethics into corporate social responsibility (CSR) initiatives can enhance a company's reputation and contribute to sustainable business practices. Companies that prioritize ethical data use are likely to gain the trust of customers, employees, and stakeholders. In the long run, this can lead to stronger customer loyalty, better employee retention, and increased investor confidence.
The Future of Ethical Data Analytics
As technology continues to evolve, the ethical landscape of data analytics will become even more complex. Emerging technologies such as artificial intelligence (AI), machine learning, and big data offer unprecedented opportunities for growth, but they also raise new ethical questions. Organizations must remain vigilant in ensuring that their use of these technologies aligns with ethical principles and that their data governance practices evolve alongside technological advancements.
Building Ethical AI Systems
To ensure the ethical use of AI in data analytics, companies must prioritize transparency, fairness, and accountability in the design and implementation of AI systems. AI algorithms should be explainable and interpretable, allowing stakeholders to understand how decisions are made. Additionally, organizations should establish mechanisms for identifying and rectifying errors or biases in AI-driven analytics.
Continuous Ethical Education and Training
Lastly, companies must invest in the continuous education of their employees on ethical issues related to data analytics. Providing regular training on privacy laws, data protection, and ethical decision-making will ensure that employees are equipped to navigate the ethical challenges that arise in their work.
Conclusion
The ethical implications of data analytics are vast and multifaceted, requiring organizations to take a proactive, transparent, and accountable approach. By adhering to principles of data privacy, bias mitigation, and ethical governance, businesses can leverage data for innovation while protecting individual rights. As we move forward into an increasingly data-driven world, it is crucial that ethics remain at the forefront of data analytics practices, ensuring that technology serves humanity in a fair and equitable manner. For those looking to better understand these ethical challenges, Offline Data Analytics Training in Noida, Delhi, Indore, Meerut and more cities in India offers valuable opportunities to gain hands-on experience and insight into responsible data practices while staying updated on the latest developments in the field.
4o