Highlights:
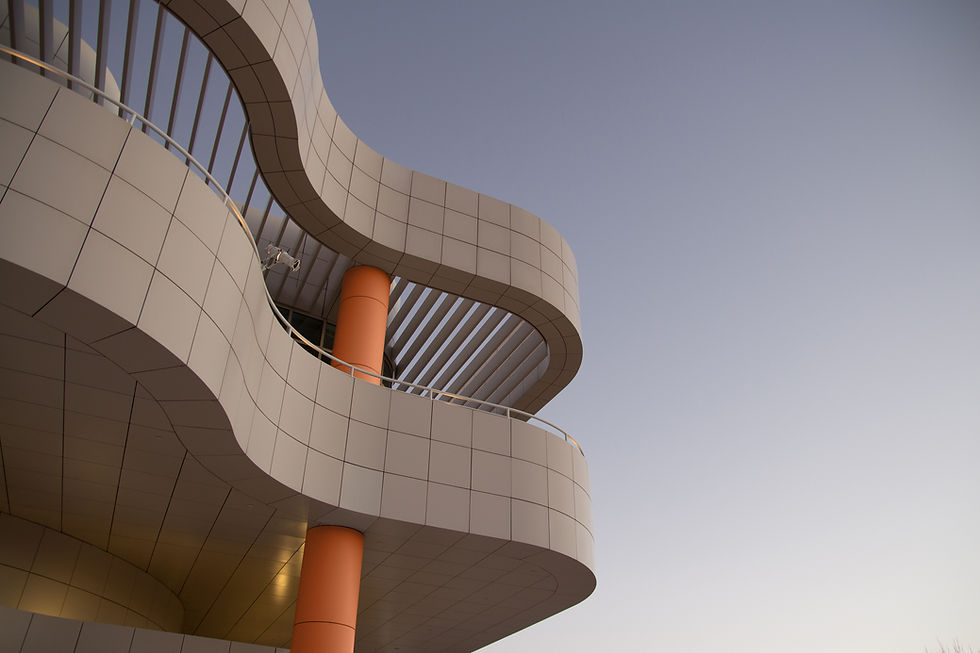
Exploring the Ethical Implications of Data Science
Oct 1, 2024
3 min read
0
3
0
In an era where data drives decisions, the field of data science has emerged as a pivotal force in industries ranging from healthcare to finance, marketing, and beyond. While the capabilities of data science offer unprecedented opportunities for innovation and improvement, they also raise significant ethical concerns. This article explores the ethical implications of data science, focusing on issues such as privacy, bias, transparency, and accountability.
The Power and Responsibility of Data Science
Data science encompasses the extraction of insights and knowledge from structured and unstructured data. With the ability to analyze vast amounts of information, data scientists can uncover patterns, predict outcomes, and inform strategic decisions. However, with great power comes great responsibility. The ethical implications of data science extend beyond mere compliance with regulations; they involve a commitment to protecting individual rights, promoting fairness, and ensuring the integrity of processes.
1. Privacy Concerns
One of the most pressing ethical issues in data science is privacy. As organizations collect more data, including personal information, the potential for misuse increases. Here are some key considerations:
Data Collection: How is data being collected? Organizations must be transparent about their data collection practices and ensure that they have the consent of individuals whose data is being used.
Data Storage and Security: Ensuring that data is stored securely and protected from breaches is essential. A lack of security measures can lead to unauthorized access, resulting in personal data exposure.
Data Usage: Organizations should be clear about how they intend to use the data collected. Unintended consequences can arise if data is used for purposes not originally disclosed to individuals.
2. Bias and Fairness
Data science relies heavily on algorithms and models, which can inadvertently perpetuate biases present in the training data. This raises ethical concerns regarding fairness:
Algorithmic Bias: If the data used to train models reflects societal biases (e.g., racial, gender, or socioeconomic biases), the outcomes produced by these models may reinforce existing inequalities. This is particularly concerning in areas such as hiring, law enforcement, and lending.
Diversity in Data: Ensuring diverse representation in training data is crucial. Data scientists must be vigilant in selecting datasets that accurately represent the population to mitigate bias.
Evaluation Metrics: Using fair evaluation metrics can help identify and address bias in algorithms. Data scientists should prioritize fairness alongside accuracy when developing models.
3. Transparency and Accountability
Transparency in data science processes is vital for building trust among stakeholders. Organizations must ensure accountability in their data practices:
Explainability: Complex algorithms can be challenging to understand. Providing clear explanations of how models work and the factors influencing their decisions is essential for transparency.
Documentation: Maintaining thorough documentation of data sources, methodologies, and assumptions can help ensure accountability. This allows stakeholders to understand the decision-making process and hold organizations accountable for their outcomes.
Stakeholder Involvement: Engaging stakeholders in the data science process can enhance transparency. Involving diverse perspectives can lead to more ethical outcomes and help identify potential ethical concerns early on.
4. The Role of Regulation and Governance
As the ethical implications of data science become more apparent, the need for robust regulatory frameworks is increasingly recognized:
Data Protection Regulations: Laws such as the General Data Protection Regulation (GDPR) and the California Consumer Privacy Act (CCPA) provide guidelines for data collection, storage, and usage, promoting ethical data practices.
Ethical Guidelines: Organizations can develop their own ethical guidelines for data science practices, outlining principles for responsible data use and the treatment of individuals' data.
Independent Oversight: Establishing independent oversight committees can help monitor data practices, ensuring compliance with ethical standards and regulations.
5. Education and Training
Education plays a crucial role in fostering ethical awareness among data scientists:
Ethics in Curriculum: Incorporating ethical considerations into data science education can equip future data scientists with the knowledge needed to navigate ethical dilemmas.
Continuous Learning: Providing ongoing training in ethical data practices can help data professionals stay informed about emerging ethical challenges and best practices.
Conclusion
As data science continues to evolve, so too must our understanding of its ethical implications. By addressing issues of privacy, bias, transparency, and accountability, data scientists can ensure that their work contributes positively to society. Emphasizing ethical considerations in data practices not only protects individuals but also fosters trust and integrity within the field. Ultimately, embracing ethical principles in data science is not just a regulatory requirement; it is a moral obligation that can lead to more equitable and just outcomes for all. For those interested in navigating these ethical complexities, enrolling in a Data Science Training Course in Noida, Delhi, Indore, Chennai and more cities in India can provide valuable insights and skills to promote responsible data practices.