Highlights:
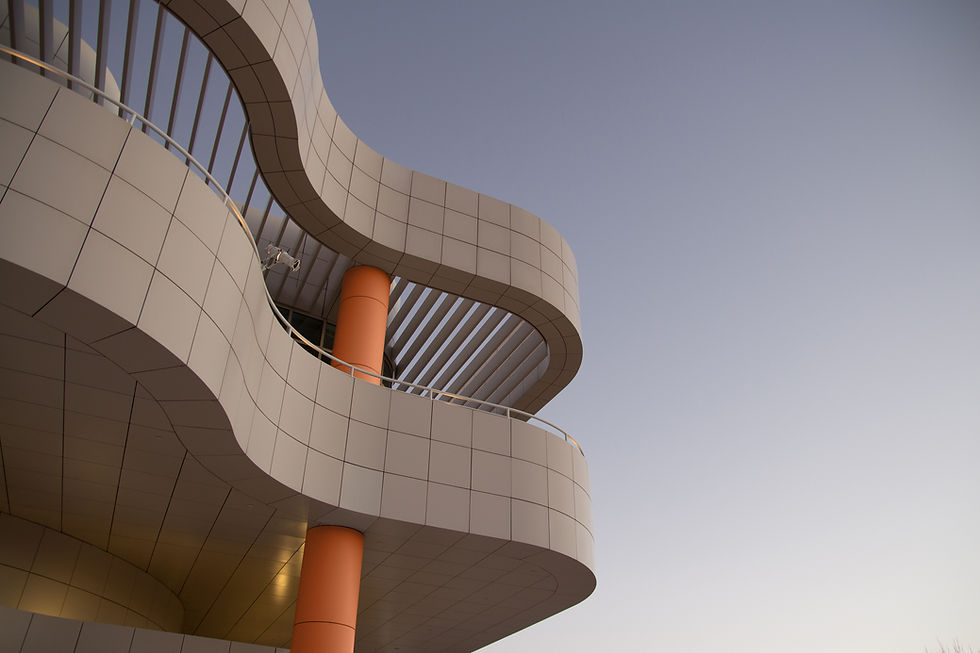
How AI is Transforming the Field of Data Analytics
2 days ago
5 min read
0
1
0
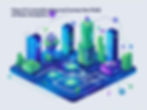
Data analytics has undergone a profound transformation, primarily fueled by the integration of Artificial Intelligence (AI). What was once a manual and time-consuming process involving spreadsheets and basic statistical tools has evolved into a sophisticated discipline driven by automation, machine learning, and advanced algorithms. The fusion of AI and data analytics is revolutionizing how organizations collect, interpret, and leverage data to make informed decisions. In this article, we will explore how AI is reshaping the field of data analytics, the technologies involved, real-world applications, challenges, and what this means for the future workforce.
Introduction to AI in Data Analytics
Artificial Intelligence refers to the simulation of human intelligence in machines that are programmed to think, learn, and make decisions. In the context of data analytics, AI involves the use of machine learning algorithms, natural language processing (NLP), and deep learning to automate data analysis tasks and uncover insights that would be difficult or impossible for humans to detect manually.
AI is not just enhancing data analytics; it is redefining it. By enabling machines to learn from vast datasets, recognize patterns, and improve over time without human intervention, AI is drastically improving the efficiency, accuracy, and scope of analytics.
The Evolution of Data Analytics
To understand the impact of AI, it’s important to recognize the evolution of data analytics over time:
Descriptive Analytics – Focused on what happened using historical data.
Diagnostic Analytics – Explains why something happened.
Predictive Analytics – Uses models to forecast future outcomes.
Prescriptive Analytics – Suggests actions based on predictions.
Cognitive Analytics (AI-driven) – Mimics human reasoning by interpreting data contextually and adapting over time.
AI empowers organizations to move beyond descriptive and diagnostic analytics toward predictive and prescriptive insights, which are highly valuable in decision-making.
Key AI Technologies in Data Analytics
a. Machine Learning (ML)
Machine learning is the backbone of AI in data analytics. It enables systems to automatically learn from data and improve their performance over time. ML algorithms are used to build predictive models, classify data, cluster similar groups, and detect anomalies.
b. Natural Language Processing (NLP)
NLP allows machines to understand and interpret human language. In data analytics, NLP is used for sentiment analysis, chatbots, automated report generation, and text mining.
c. Deep Learning
Deep learning involves neural networks with many layers that can learn complex patterns in large amounts of data. It is used in image recognition, voice processing, and advanced predictive modeling.
d. Robotic Process Automation (RPA)
RPA combined with AI automates repetitive, rule-based tasks in data collection, data cleaning, and reporting. It reduces manual labor and improves productivity.
AI in the Data Analytics Workflow
Let’s look at how AI is applied throughout the data analytics workflow:
a. Data Collection and Integration
AI-powered tools can automatically extract, transform, and load data from multiple sources such as websites, databases, social media platforms, and IoT devices. This reduces the time spent on data preparation.
b. Data Cleaning and Preprocessing
AI algorithms can identify and correct inconsistencies, missing values, and outliers. Intelligent preprocessing ensures that the data fed into analytics models is clean and reliable.
c. Data Analysis and Modeling
AI enables the development of complex models that go beyond traditional statistical analysis. Machine learning models can predict trends, identify customer behavior, detect fraud, and more with high accuracy.
d. Visualization and Reporting
With the help of NLP and AI, dashboards can now auto-generate insights and reports using plain English summaries. AI-driven visualization tools can highlight key patterns and suggest areas that need attention.
Real-World Applications of AI in Data Analytics
a. Healthcare
AI helps in analyzing patient data to predict disease outbreaks, recommend treatments, and optimize hospital resources. Predictive analytics aids in early diagnosis and personalized medicine.
b. Finance
In the financial sector, AI is used for fraud detection, credit scoring, risk assessment, and algorithmic trading. It processes transactions in real-time and flags suspicious activities.
c. Retail and E-commerce
Retailers use AI to analyze customer behavior, personalize recommendations, optimize pricing strategies, and manage inventory. Chatbots powered by AI also enhance customer service.
d. Marketing
Marketing teams use AI to segment audiences, predict campaign performance, and automate email targeting. Sentiment analysis from social media gives brands real-time customer feedback.
e. Manufacturing
In manufacturing, AI-driven predictive maintenance helps in reducing downtime and optimizing the use of machinery. AI also aids in quality control through image recognition.
Benefits of AI in Data Analytics
Speed and Efficiency: AI can process large datasets much faster than humans, allowing organizations to make timely decisions.
Accuracy: Automated systems reduce the risk of human error in data handling and interpretation.
Scalability: AI systems can handle growing volumes of data without performance drops.
Predictive Power: Advanced AI models can forecast trends and behaviors with a high degree of confidence.
Cost Reduction: By automating repetitive tasks, organizations save on manpower and operational costs.
Challenges and Limitations
Despite its advantages, the integration of AI in data analytics comes with certain challenges:
Data Privacy and Ethics: Using AI on personal data raises concerns about privacy and compliance with regulations like GDPR.
Bias in Algorithms: AI systems are only as unbiased as the data they are trained on. Poor data can lead to skewed results.
Lack of Skilled Professionals: There’s a growing demand for professionals who understand both AI and analytics.
Integration with Legacy Systems: Many organizations struggle to integrate AI tools with their existing infrastructure.
Transparency: Some AI models, especially deep learning, are seen as “black boxes,” making it hard to explain decisions.
The Future of Data Analytics with AI
The future of data analytics lies in even deeper AI integration. Here’s what we can expect:
Automated Analytics: Tools that not only analyze data but also generate insights and take action automatically.
Augmented Analytics: AI will work alongside humans, enhancing their capabilities rather than replacing them.
Edge Analytics: AI-powered devices will analyze data at the edge (e.g., sensors, cameras) without sending it to the cloud.
Conversational Analytics: Voice and text interfaces that allow users to query data in natural language.
AI Democratization: More user-friendly tools will enable non-technical users to harness the power of AI analytics.
Career Opportunities in AI-Powered Data Analytics
As AI becomes integral to data analytics, new career opportunities are emerging:
AI Data Analyst
Machine Learning Engineer
Data Scientist
Data Engineer
Analytics Consultant
Business Intelligence Analyst
To prepare for these roles, professionals need to upskill in areas like machine learning, Python/R programming, and data visualization tools. Fortunately, educational institutions are adapting to this demand. For example, those looking to develop both foundational and advanced analytics skills can benefit from a data analytics training in Delhi, Lucknow Meerut and more cities in India where structured programs are tailored to meet the needs of today’s AI-driven analytics landscape.
Conclusion
AI is not just a trend in data analytics—it’s a revolution. From automating tasks to delivering deeper insights, AI is transforming every aspect of how data is understood and used. While challenges remain, the potential for innovation, efficiency, and smarter decision-making is immense. As we move forward, the synergy between human intelligence and artificial intelligence will be the cornerstone of impactful analytics.
For professionals and organizations alike, embracing this transformation is not optional—it’s essential. Investing in AI-powered analytics tools, upskilling through courses, and fostering a culture of data-driven thinking will define success in the coming decades.