Highlights:
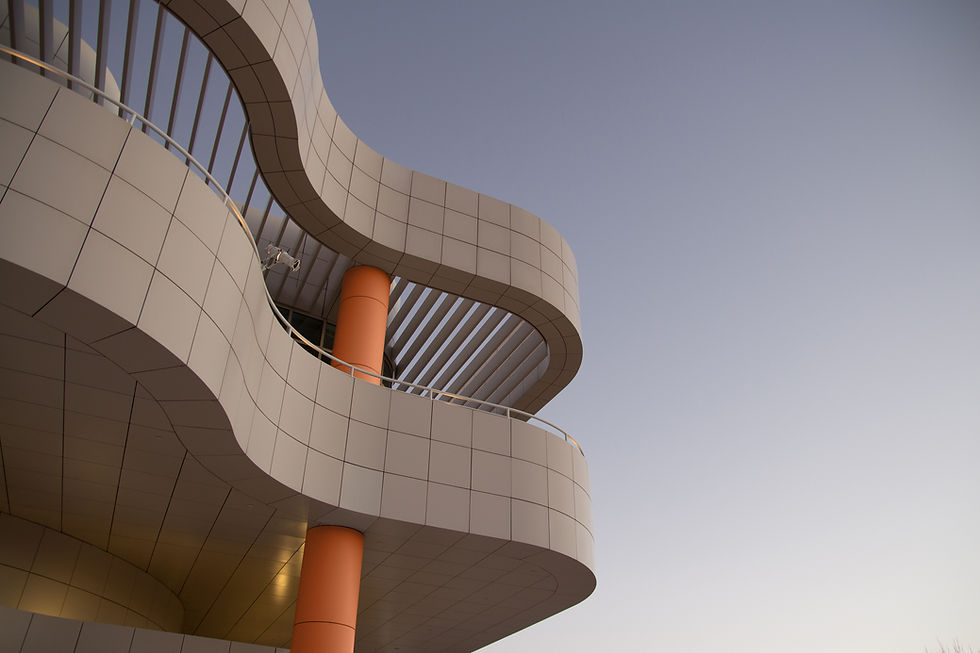
How Data Science Is Powering Smart Cities and IoT
4 days ago
5 min read
0
2
0
Introduction
In the age of digital transformation, cities around the world are becoming smarter, safer, and more efficient through the integration of cutting-edge technologies. At the heart of this evolution lies data science, a powerful discipline that enables real-time decision-making, predictive analytics, and automation. Coupled with the Internet of Things (IoT)—a vast network of interconnected devices—data science is revolutionizing urban life. From traffic control and energy efficiency to waste management and public safety, the synergy of data science and IoT is driving the smart city revolution.
This article explores how data science is fueling smart city initiatives, its connection with IoT, the benefits, the challenges, and what the future holds.
What Are Smart Cities?
A smart city uses digital technology and data to enhance the quality of life for its citizens. It involves deploying sensors, collecting and analyzing data, and using insights to improve urban services and infrastructure. Smart cities are built on key pillars such as:
Smart governance
Smart transportation
Smart energy
Smart buildings
Smart healthcare
Smart environment
These components are driven by data generated from millions of connected devices and systems, which must be analyzed and interpreted efficiently—enter data science.
The Role of IoT in Smart Cities
The Internet of Things (IoT) refers to the network of physical objects embedded with sensors, software, and other technologies to connect and exchange data with other devices and systems over the internet. In a smart city context, IoT includes:
Smart meters
Surveillance systems
Smart lighting
Traffic signals
Air quality sensors
Connected vehicles
Waste monitoring bins
These devices generate massive amounts of data every second. This is where data science becomes crucial—transforming raw data into actionable insights.
How Data Science Works with IoT in Smart Cities
Data collected through IoT devices is often high in volume, velocity, and variety—the classic “3Vs” of Big Data. Data scientists apply analytical techniques and algorithms to extract meaningful insights from this raw data. Here’s how the integration works:
Data Collection: IoT devices capture real-time data from city infrastructure.
Data Transmission: Data is transmitted securely through communication networks.
Data Processing: Data science algorithms process and clean the data.
Analytics & Machine Learning: Predictive models are applied to identify patterns, trends, and anomalies.
Decision Making: Actionable insights are used by city officials to optimize services.
Use Cases: How Data Science Powers Smart Cities
1. Smart Traffic Management
Congested roads are a daily challenge in urban areas. IoT sensors and cameras monitor traffic flow, while data science algorithms analyze the data to:
Predict traffic congestion
Optimize signal timings
Suggest alternate routes
Plan road expansions
Example: Cities like Barcelona and Singapore use predictive analytics to reduce congestion and commute times.
2. Energy Efficiency and Smart Grids
Smart grids use real-time data to balance supply and demand for electricity. Data science:
Forecasts energy consumption
Detects inefficiencies
Automates power distribution
Smart thermostats and meters also help homeowners reduce bills while contributing to sustainable energy use.
3. Waste Management Optimization
Overflowing bins and inefficient routes waste time and fuel. Smart waste bins send data on their fill levels, and machine learning models plan optimal collection routes. This ensures cleaner streets and reduced emissions.
4. Environmental Monitoring
Smart cities deploy IoT sensors to monitor air and water quality. Data science models track pollution levels, identify sources, and predict hazardous trends. This data aids in policy decisions and public health alerts.
5. Public Safety and Crime Prediction
CCTV, drones, and social media data are combined to enhance surveillance. Predictive policing uses data science to forecast crime hotspots, allowing law enforcement to act proactively.
6. Smart Parking
Sensors detect available parking spaces and share this data with users through mobile apps. Data science helps optimize parking distribution and predict peak hours, reducing traffic and frustration.
7. Healthcare and Emergency Response
IoT-enabled wearable devices monitor vital signs and alert emergency services in case of anomalies. Data science models analyze historical and real-time health data to prioritize emergency response and allocate resources.
The Role of AI and Machine Learning
AI and machine learning are integral parts of data science and enhance the performance of smart city systems. Key applications include:
Predictive Maintenance: Identifying equipment failure in public infrastructure before it happens.
Natural Language Processing (NLP): Analyzing citizen feedback and social media to understand public sentiment.
Computer Vision: Enhancing surveillance with facial recognition and object detection.
Anomaly Detection: Spotting irregularities in water usage or network activity for early intervention.
Challenges in Implementing Data Science in Smart Cities
Despite the immense potential, several challenges exist:
1. Data Privacy and Security
Handling vast amounts of personal and location-based data raises concerns about surveillance and misuse. Robust encryption and regulatory frameworks are necessary.
2. Data Integration
Smart cities involve multiple data sources—government, private sector, sensors—which can create silos. Ensuring compatibility and integration is a major hurdle.
3. Infrastructure and Investment
Developing a fully functional smart city demands substantial investment in digital infrastructure and skilled personnel.
4. Skilled Workforce
Data science talent is essential for smart city success. Professionals must be trained in data analytics, machine learning, and urban planning.
The Economic and Social Impact
The implementation of data science in smart cities has a ripple effect:
Cost Reduction: Efficient resource management saves public money.
Job Creation: New opportunities arise in data engineering, urban analytics, and AI development.
Improved Quality of Life: Citizens enjoy cleaner air, safer streets, and efficient services.
Sustainability: Smart cities are more aligned with sustainable development goals (SDGs).
Global Examples of Smart Cities Using Data Science
1. Singapore: Uses data to control traffic lights, predict maintenance needs, and provide real-time transport updates.
2. Barcelona: Leverages data to optimize energy use, manage waste, and engage citizens via smart apps.
3. Amsterdam: Applies open data platforms to crowdsource solutions to urban issues and make services transparent.
4. Dubai: Using AI-powered data analytics, Dubai aims to become one of the most connected cities in the world by 2030.
Learning Data Science to Contribute to Smart Cities
As the demand for smarter infrastructure grows, so does the need for skilled data science professionals. If you're inspired to contribute to this transformation, pursuing a data science training course in Noida, Delhi, Lucknow, Meerut, and more cities in India can be your first step. Programs like these offer hands-on experience in Python, machine learning, big data tools, and cloud computing, preparing learners for real-world smart city projects.
The Future of Smart Cities and Data Science
Looking ahead, the fusion of 5G, edge computing, blockchain, and AI will take smart cities to the next level. Imagine a city that:
Self-heals its infrastructure
Prevents accidents before they occur
Automatically reroutes traffic during emergencies
Balances its energy needs in real time
These aren’t dreams—they’re in development right now, thanks to data science and IoT.
Conclusion
Data science and IoT are not just buzzwords—they are the backbone of modern smart cities. With the power to improve services, protect the environment, and make urban life safer and more convenient, the synergy between these technologies is shaping the cities of tomorrow. Whether you're a policymaker, a tech enthusiast, or an aspiring data scientist, understanding this revolution is the first step to becoming part of it.