Highlights:
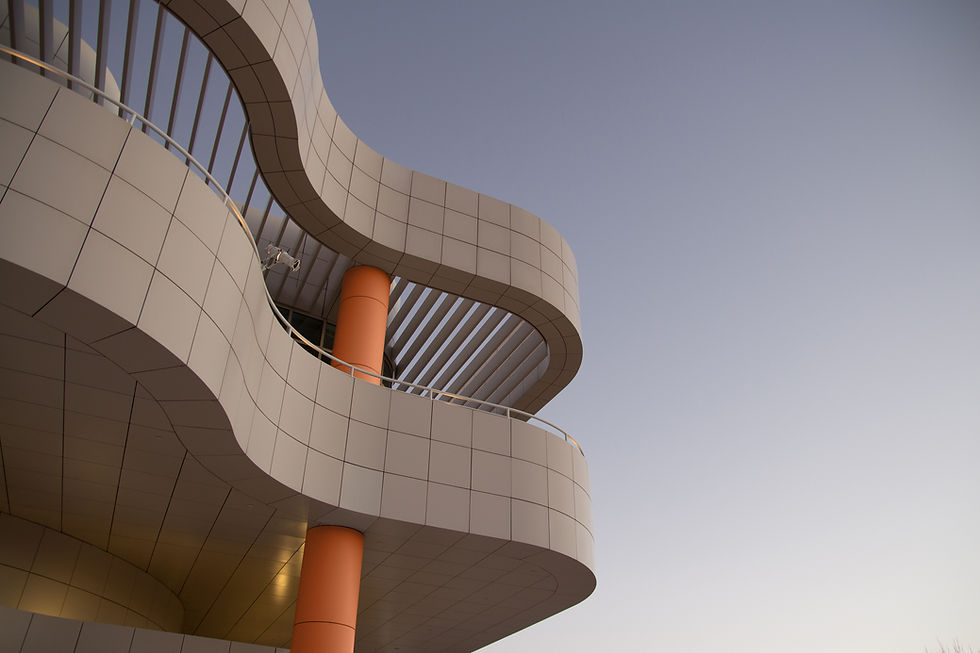

Introduction
With data science harnessing the power of data for predictive modeling, automation, and decision-making, the landscape of business operations has significantly transformed. However, as the volume and sensitivity of data grow, so do concerns about data privacy and protection. This is where the General Data Protection Regulation (GDPR) becomes a pivotal consideration for data scientists, analysts, and organizations that handle personal data.
The GDPR, introduced by the European Union, was designed to enforce strict guidelines on data usage, giving individuals more control over their personal information. For data science professionals, this regulation not only presents compliance challenges but also calls for a re-evaluation of methodologies, tools, and ethical considerations. This article delves deeply into how GDPR affects data science, its challenges, and the best practices to align data strategies with privacy laws.
GDPR: Scope and Objectives
The General Data Protection Regulation (GDPR) came into force on May 25, 2018, and applies to all organizations processing personal data of individuals residing in the European Union, regardless of where the organization is based. The regulation is built upon several key principles:
Lawfulness, fairness, and transparency
Purpose limitation
Data minimization
Accuracy
Storage limitation
Integrity and confidentiality
Accountability
GDPR aims to ensure that individuals understand how their data is being used and to empower them with rights such as the right to access, rectify, delete, and restrict the use of their data.
Data Science Before GDPR: The Wild West Era
Before GDPR, data science practices operated in a relatively unregulated space. Organizations collected massive amounts of personal data through cookies, mobile apps, social platforms, and online transactions, often without explicit user consent. This data was then used to train machine learning models, run sentiment analysis, or create targeted marketing campaigns.
The lack of regulation meant that data scientists had wide freedom in collecting and using data. While this fostered innovation, it also raised ethical concerns around data exploitation, profiling, and surveillance. GDPR came as a necessary intervention to ensure a balance between innovation and individual rights.
Key Ways GDPR Impacts Data Science
1. Consent Management Under GDPR, organizations must obtain clear, informed consent from individuals before collecting or processing their data. This has significant implications for data science:
Data cannot be used for purposes beyond what the user has consented to.
Consent must be granular, meaning users should be able to choose what types of data they agree to share.
Consent must be documented and revocable at any time.
2. Data Minimization The principle of data minimization restricts data collection to only what is necessary for a specific purpose. This challenges traditional data science methodologies that rely on collecting extensive datasets to improve model accuracy.
3. Anonymization and Pseudonymization To reduce risks, GDPR encourages anonymizing or pseudonymizing personal data.
Anonymization: Data is stripped of identifiers, making it impossible to trace back to an individual.
Pseudonymization: Identifiers are replaced with pseudonyms or codes, and the key is kept separately.
These techniques protect individual privacy but may limit the depth of analysis possible in some data science projects.
4. Right to Be Forgotten: Individuals can request the deletion of their data. For data science, this implies:
The need to track and isolate user data across datasets.
Potential re-training of models if the deleted data was part of a training set.
5. Data Portability Users can request their data in a machine-readable format. Data scientists must ensure that data is stored in formats that are transferable and standardized.
6. Algorithmic Transparency and Fairness GDPR mandates that individuals have the right to understand how automated decisions affecting them are made. This affects black-box models that lack explainability.
Technical and Organizational Challenges
1. Model Reengineering Removing data subjects from models can necessitate re-training, especially if their data significantly influenced the model. This adds resource and cost implications.
2. Data Governance Frameworks Organizations must now have clear policies on data storage, access, sharing, and lifecycle management. This demands coordination between data scientists, legal teams, and IT departments.
3. Reduced Data Access With stricter consent and minimization policies, data scientists often have access to smaller datasets, potentially impacting the effectiveness of machine learning models.
4. Balancing Utility and Privacy Implementing privacy-preserving techniques like differential privacy, federated learning, and synthetic data generation is complex but necessary.
Privacy-Preserving Techniques in Data Science
1. Differential Privacy adds noise to datasets to prevent the identification of individuals while maintaining overall trends.
2. Federated Learning allows models to be trained across multiple decentralized devices or servers holding local data samples, without exchanging data.
3. Homomorphic Encryption enables computations to be performed on encrypted data without decrypting it first.
4. Synthetic Data Generation creates artificial datasets that mimic the statistical properties of real data without containing actual personal data.
These techniques help maintain data utility while ensuring compliance with GDPR.
Ethical Considerations and Accountability
GDPR has also placed a spotlight on the ethical responsibilities of data scientists. Key considerations include:
Bias Mitigation: Ensuring models do not reinforce existing societal biases.
Transparency: Making AI systems understandable to non-technical stakeholders.
Human Oversight: Maintaining human involvement in critical decision-making processes.
Preparing for GDPR as a Data Scientist
Professionals entering the data science field need to be equipped not just with technical skills but also with legal awareness and ethical sensitivity. This is why many educational programs, such as a data science training course in Delhi, Noida, Lucknow, Meerut, Indore, and more cities in India now include modules on data ethics, GDPR compliance, and responsible AI practices.
Key learning objectives should include:
Understanding GDPR principles
Knowing how to handle data subject requests
Learning to implement anonymization and pseudonymization
Gaining familiarity with privacy-preserving ML techniques
Building transparent and interpretable models
Organizational Strategies for GDPR Compliance
Organizations can support data scientists in achieving GDPR compliance through:
Data Mapping and Classification: Identifying where personal data is stored and how it flows across systems.
Data Protection Officers (DPOs): Appointing DPOs to oversee compliance.
Data Subject Request Management: Creating efficient workflows to handle access, deletion, and portability requests.
Audit Trails: Maintaining logs for data access, modification, and sharing.
The Global Influence of GDPR
GDPR has set a benchmark that has inspired similar regulations worldwide:
CCPA (California Consumer Privacy Act) in the United States
PIPEDA (Personal Information Protection and Electronic Documents Act) in Canada
PDPB (Personal Data Protection Bill) in India
These laws mirror GDPR in many ways, suggesting a global shift toward data rights and privacy.
Conclusion
GDPR has fundamentally changed the way data science is practiced. While it introduces operational complexities and limitations, it also fosters a more ethical and accountable approach to data analysis. In this evolving landscape, data scientists must adapt by learning new tools, methods, and legal frameworks.
By aligning innovation with responsibility, data science can continue to thrive in a privacy-conscious world.