Highlights:
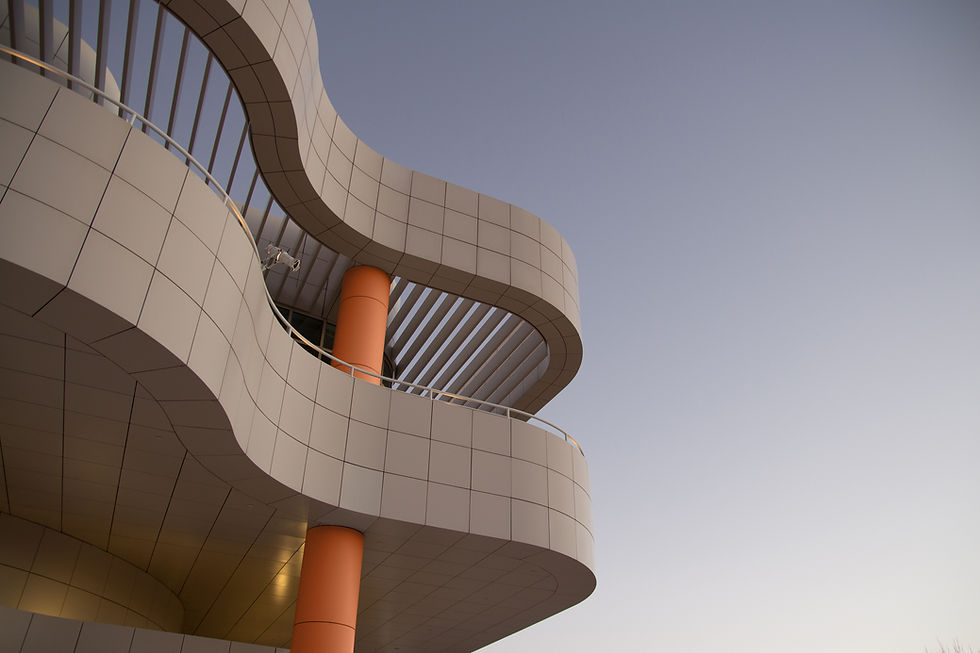
The Role of Data Analytics in Higher Education
Sep 26, 2024
7 min read
0
3
0
Data analytics is increasingly becoming a vital component of various sectors, and higher education is no exception. As institutions strive to enhance student outcomes, improve operational efficiency, and stay competitive in a rapidly evolving educational landscape, the role of data analytics is more crucial than ever. This article delves into the multifaceted impact of data analytics in higher education, highlighting its benefits, applications, challenges, and future prospects.
Understanding Data Analytics in Higher Education
Data analytics refers to the systematic computational analysis of data. In higher education, it involves collecting, processing, and interpreting data from various sources, such as student information systems, learning management systems, and external data sets. The goal is to extract meaningful insights that can inform decision-making and drive improvements in academic and administrative processes.
Types of Data Analytics
Data analytics can be categorized into three main types:
Descriptive Analytics: This type focuses on summarizing historical data to understand what has happened. For example, universities can analyze student enrollment trends over the years to gauge the popularity of certain programs.
Predictive Analytics: This involves using statistical models and machine learning techniques to forecast future outcomes based on historical data. For instance, institutions can predict student retention rates by analyzing past behaviors and demographics.
Prescriptive Analytics: This type provides recommendations for actions based on data analysis. For example, data analytics can help institutions identify which interventions are most likely to improve student success.
Enhancing Student Success
Personalized Learning Experiences
One of the primary applications of data analytics in higher education is to enhance student success through personalized learning experiences. By analyzing student data, institutions can identify individual learning styles, preferences, and challenges. This allows educators to tailor their teaching methods and resources to meet the specific needs of each student.
For example, learning management systems can track student engagement and performance metrics, enabling instructors to intervene when a student is struggling. Additionally, adaptive learning technologies can adjust the difficulty of assignments based on a student’s progress, ensuring that they are always challenged without becoming overwhelmed.
Identifying At-Risk Students
Data analytics plays a critical role in identifying at-risk students before they disengage or drop out. By analyzing various factors, such as attendance, grades, and participation in extracurricular activities, institutions can pinpoint students who may need additional support.
Once identified, universities can implement targeted interventions, such as tutoring programs, mentoring, or counseling services. Research has shown that early intervention can significantly improve retention rates and help students succeed academically.
Improving Academic Advising
Data analytics can also enhance academic advising by providing advisors with insights into students’ academic histories and career aspirations. By analyzing course performance, major choices, and career trajectories, advisors can offer more informed guidance to students.
For instance, if a student is struggling in a particular subject, the advisor can recommend relevant resources or alternative courses that align with the student’s strengths and interests. This data-driven approach leads to more effective academic planning and better outcomes for students.
Optimizing Operational Efficiency
Streamlining Admissions Processes
Data analytics can significantly improve the efficiency of admissions processes. By analyzing historical admissions data, institutions can identify trends in applicant demographics, qualifications, and enrollment patterns. This information enables universities to refine their recruitment strategies and allocate resources more effectively.
For example, by understanding which recruitment channels yield the highest-quality applicants, institutions can focus their efforts on those areas. Additionally, predictive analytics can help institutions forecast enrollment numbers, allowing them to plan accordingly for staffing, housing, and resources.
Enhancing Resource Allocation
Institutions can leverage data analytics to optimize resource allocation across various departments. By analyzing operational data, universities can identify areas where resources are underutilized or overextended. This analysis can inform decisions regarding budget allocation, staffing, and facility management.
For instance, if data reveals that certain programs are consistently oversubscribed while others remain underenrolled, institutions can adjust course offerings or reallocate funding to enhance academic offerings.
Supporting Financial Planning
Financial sustainability is a major concern for many higher education institutions. Data analytics can aid in financial planning by providing insights into revenue streams, expenses, and financial aid distribution. By analyzing financial data, universities can identify areas for cost savings and explore new funding opportunities.
For example, institutions can analyze the effectiveness of different fundraising campaigns or grant applications, allowing them to allocate resources more effectively and increase financial support for critical programs.
Enhancing Research and Innovation
Supporting Institutional Research
Data analytics empowers institutions to conduct more effective institutional research. By analyzing data on student performance, faculty effectiveness, and program outcomes, institutions can assess the impact of their initiatives and policies. This data-driven approach allows for continuous improvement and informed decision-making.
For example, by analyzing graduation rates and post-graduation outcomes, institutions can evaluate the effectiveness of specific programs and make necessary adjustments to enhance student success.
Fostering Collaborative Research
Data analytics also plays a significant role in fostering collaborative research among institutions. By sharing data and insights, universities can work together on research projects, grant applications, and joint initiatives. This collaboration can lead to more innovative solutions to pressing educational challenges.
Additionally, partnerships with industry can be strengthened through data analytics. By analyzing labor market data, institutions can align their academic programs with industry needs, ensuring that graduates possess the skills and knowledge demanded by employers.
Challenges of Data Analytics in Higher Education
While data analytics offers numerous benefits, several challenges must be addressed for its effective implementation in higher education.
Data Privacy and Security
One of the primary concerns surrounding data analytics is the privacy and security of student information. Institutions must ensure that they comply with legal and ethical standards when collecting, storing, and analyzing data. Implementing robust data protection measures is crucial to safeguard sensitive information and maintain student trust.
Data Quality and Integration
The effectiveness of data analytics hinges on the quality and integration of data. Institutions often collect data from various sources, which can lead to inconsistencies and gaps. Ensuring data accuracy and reliability is essential for meaningful analysis. Additionally, integrating data across different systems can be complex and time-consuming.
Resistance to Change
The adoption of data analytics may face resistance from faculty and staff who are accustomed to traditional methods. Institutions must foster a culture of data-driven decision-making and provide adequate training and support to facilitate the transition. Engaging stakeholders in the process and demonstrating the benefits of data analytics can help mitigate resistance.
Future Prospects of Data Analytics in Higher Education
The future of data analytics in higher education is promising, with several emerging trends poised to shape its evolution.
Increased Use of Artificial Intelligence
As technology continues to advance, the integration of artificial intelligence (AI) and machine learning into data analytics will become more prevalent. These technologies can enhance predictive analytics capabilities, allowing institutions to make even more accurate forecasts and informed decisions.
For example, AI algorithms can analyze vast amounts of data to identify patterns and correlations that may not be evident to human analysts. This can lead to more effective interventions and improved student outcomes.
Enhanced Learning Analytics
Learning analytics, a subset of data analytics focused on understanding and optimizing learning processes, will play an increasingly important role in higher education. By analyzing data from various learning platforms and tools, institutions can gain insights into student engagement, learning behaviors, and instructional effectiveness.
This information can inform the development of more effective teaching strategies and educational technologies, ultimately leading to improved learning experiences for students.
Data-Driven Institutional Strategies
As the demand for accountability and transparency in higher education grows, institutions will increasingly rely on data-driven strategies to demonstrate their effectiveness and value. Data analytics will play a crucial role in assessing program outcomes, student satisfaction, and institutional performance.
By leveraging data, institutions can make informed decisions that align with their mission and goals, ensuring that they continue to meet the evolving needs of students and society.
Conclusion
The role of data analytics in higher education is transformative, offering numerous benefits for students, faculty, and administrators alike. By harnessing the power of data, institutions can enhance student success, optimize operations, and drive innovation. For instance, Data Analytics Classes in Noida, Delhi, Indore, Mumbai, Chennai and more cities in India are equipping students with essential skills to utilize data effectively in educational settings. However, to fully realize these benefits, institutions must address the challenges associated with data privacy, quality, and integration.
As technology continues to evolve, the future of data analytics in higher education holds great promise, with the potential to shape the educational landscape for years to come. Embracing data-driven decision-making will not only enhance the quality of education but also ensure that institutions remain relevant in an ever-changing world.
FAQs
1. What is data analytics in higher education? Data analytics in higher education refers to the systematic analysis of data collected from various sources within educational institutions to improve decision-making, enhance student success, and optimize operational efficiency.
2. How can data analytics improve student success? Data analytics can improve student success by providing personalized learning experiences, identifying at-risk students, and enhancing academic advising, ultimately leading to better academic outcomes.
3. What are the main types of data analytics used in higher education? The main types of data analytics used in higher education are descriptive analytics (summarizing historical data), predictive analytics (forecasting future outcomes), and prescriptive analytics (providing recommendations for action).
4. What challenges does higher education face in implementing data analytics? Challenges include data privacy and security concerns, data quality and integration issues, and resistance to change from faculty and staff.
5. How will data analytics evolve in the future of higher education? Data analytics will evolve with increased use of artificial intelligence, enhanced learning analytics, and more data-driven institutional strategies to demonstrate effectiveness and improve educational outcomes.
6. Why is data privacy important in higher education analytics? Data privacy is crucial in higher education analytics to protect sensitive student information, comply with legal and ethical standards, and maintain trust between institutions and their students.