Highlights:
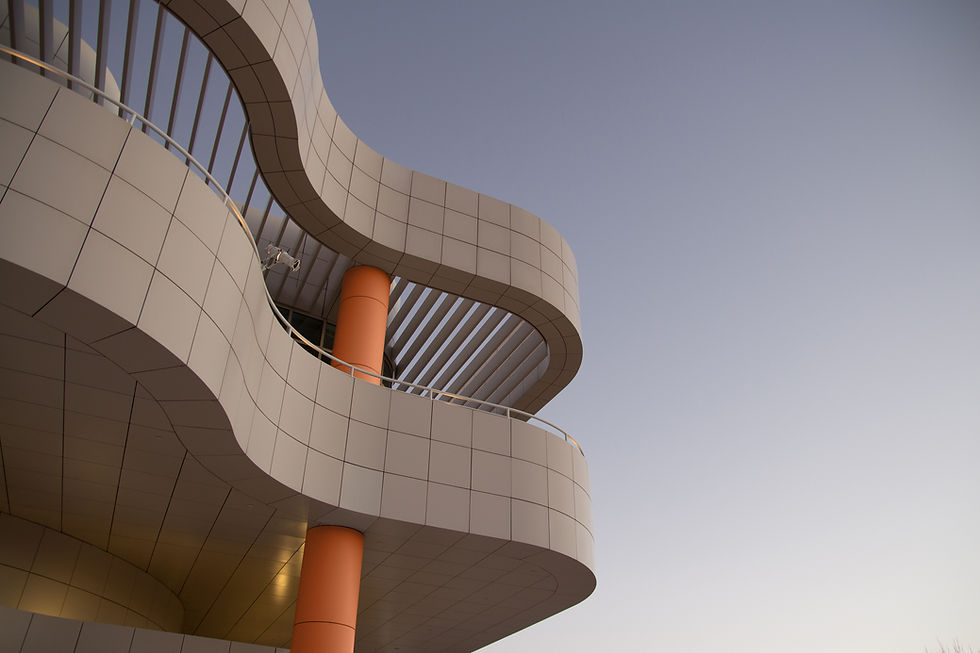
The Role of Data Science in Financial Fraud Detection
Feb 14
6 min read
0
2
0
Financial fraud is a major concern for businesses, governments, and individuals across the globe. It involves a range of deceptive activities such as identity theft, fraudulent transactions, embezzlement, insider trading, and money laundering, which can result in significant financial losses. As technology advances and financial transactions become more complex, detecting and preventing these fraudulent activities has become a growing challenge. However, thanks to the innovations in data science, financial institutions now have powerful tools and techniques to identify and mitigate fraud in real time.
Data science plays a crucial role in financial fraud detection by leveraging advanced algorithms, machine learning (ML) models, and big data analytics to identify patterns, detect anomalies, and predict potential fraudulent activities. The increasing availability of large datasets and improved computational power have made data science an indispensable tool for combating financial fraud.
Understanding Financial Fraud
Financial fraud encompasses a variety of illegal activities that exploit weaknesses within financial systems. Common forms of financial fraud include:
Credit Card Fraud: Unauthorized use of a credit card or debit card for transactions.
Identity Theft: Stealing personal information to open fraudulent accounts or commit financial crimes.
Insurance Fraud: Providing false information to claim insurance benefits.
Bank Fraud: Manipulation of banking transactions, often involving misrepresentation or deceit.
Investment Fraud: False promises of returns on investment to deceive investors.
Money Laundering: Concealing the origins of illegally obtained money, often through a series of complex transactions.
Given the range of fraudulent activities that exist, the task of detecting and preventing fraud can be incredibly challenging. Traditional methods such as manual audits and rule-based systems often fall short due to the sheer volume and complexity of financial transactions. This is where data science comes into play, offering data-driven solutions to detect and prevent fraud more effectively.
How Data Science Is Used in Financial Fraud Detection
Data science uses a variety of tools, techniques, and methodologies to help organizations detect fraudulent activities. Below are some key ways in which data science is applied in financial fraud detection:
1. Data Mining and Pattern Recognition
One of the core functions of data science is to analyze vast amounts of data to discover hidden patterns. Financial institutions process millions of transactions daily, making it difficult to spot irregularities manually. By using data mining techniques, data scientists can sift through these massive datasets to identify suspicious patterns that may indicate fraudulent activity.
For example, a sudden spike in transactions for a specific account, an increase in small transaction amounts, or unusual activity from a particular geographical region could all signal potential fraud. Machine learning algorithms can automatically flag such transactions for further investigation, making the detection process more efficient and timely.
2. Anomaly Detection
Anomaly detection is a technique used to identify unusual behavior or data points that do not conform to expected patterns. In the context of financial fraud, anomaly detection can identify transactions that are out of the ordinary, such as:
A customer suddenly makes a large withdrawal from an ATM in a foreign country.
An account showing a drastic change in transaction history, such as numerous high-value transactions occurring within a short period.
A user changes their IP address or device unexpectedly during a transaction.
Using algorithms such as clustering, classification, and regression, data scientists can build models that learn from historical transaction data and flag anomalies in real-time, reducing the risk of undetected fraud.
3. Machine Learning and Predictive Analytics
Machine learning is a subset of artificial intelligence that allows systems to learn from historical data and improve over time. In financial fraud detection, machine learning algorithms can be trained to recognize patterns of fraudulent activity based on labeled data (i.e., known cases of fraud).
The advantage of machine learning in fraud detection is that it can continuously improve its predictive accuracy as more data is fed into the model. Algorithms like decision trees, neural networks, and support vector machines can make highly accurate predictions about the likelihood of fraud. For example, an ML model can predict the probability of a particular transaction being fraudulent based on factors such as transaction history, the geographical location of the transaction, and the time of day.
4. Real-Time Fraud Detection
In the past, fraud detection systems would only analyze historical data, often leading to delayed detection of fraudulent activities. Today, data science enables real-time fraud detection, where transactions are analyzed and flagged as fraudulent as soon as they occur.
Using stream processing and big data technologies, financial institutions can process and analyze vast amounts of data in real-time. This enables immediate intervention when a potential fraud is detected, preventing further financial loss. For example, if a bank notices that a customer's credit card is being used in multiple high-value transactions across different locations in a short period, the transaction can be automatically declined, and the customer can be notified instantly.
5. Natural Language Processing (NLP)
Natural Language Processing (NLP) is another area where data science is making strides in fraud detection. NLP techniques allow computers to understand, interpret, and generate human language. In the context of fraud detection, NLP can be applied to analyze unstructured data, such as emails, chat logs, and customer complaints, to identify potential fraudulent behavior.
For instance, NLP can be used to analyze customer complaints for signs of fraudulent activity or to detect phishing attempts by analyzing the language used in fraudulent emails or messages.
6. Social Network Analysis
Financial fraud is not always committed by a single individual. Often, fraudsters work in groups or have a network of accomplices. Social network analysis, a technique in data science, helps identify connections and relationships within networks that might indicate coordinated fraudulent behavior.
By analyzing transactional data, communication patterns, and social interactions, data scientists can identify suspicious groups or clusters of individuals involved in fraudulent schemes. This approach has proven effective in detecting organized financial fraud, such as money laundering and insider trading.
The Impact of Data Science on Financial Fraud Detection
The integration of data science into financial fraud detection has led to several significant benefits:
Increased Accuracy: Data science techniques, particularly machine learning, have greatly improved the accuracy of fraud detection systems. These systems can identify suspicious activities with a higher level of precision, reducing the number of false positives and ensuring that legitimate transactions are not flagged unnecessarily.
Faster Detection and Response: With real-time analysis and predictive analytics, fraud can be detected and addressed almost immediately, reducing the potential damage caused by fraudulent transactions.
Cost-Effectiveness: Automated fraud detection systems powered by data science are more efficient and cost-effective than manual processes. Financial institutions can save resources while improving the overall security of their systems.
Improved Customer Trust: By actively detecting and preventing fraud, financial institutions can provide a safer environment for their customers, thereby improving customer trust and loyalty.
Adaptability to New Threats: Fraud tactics are constantly evolving, and traditional fraud detection systems can struggle to keep up. However, data science techniques such as machine learning allow systems to adapt and improve over time, making them more resilient to new types of fraud.
The Future of Financial Fraud Detection
As technology continues to advance, data science will play an even larger role in financial fraud detection. Innovations such as blockchain, artificial intelligence, and advanced machine learning techniques will further enhance the ability of financial institutions to detect and prevent fraud.
Furthermore, as the amount of financial data grows, financial institutions will increasingly rely on sophisticated algorithms and AI to process this data quickly and accurately. The use of advanced techniques such as deep learning and reinforcement learning will likely result in even more effective fraud detection systems.
How to Get Started in Data Science for Financial Fraud Detection
As the demand for data science professionals in the financial sector continues to grow, individuals interested in pursuing a career in financial fraud detection can benefit from formal training and education. One way to get started is by enrolling in an Online Data Science Course in Noida, Delhi, Lucknow, Meerut, Indore, and more cities in India. These courses offer comprehensive training in data analysis, machine learning, statistics, and programming, providing the essential skills needed to tackle complex problems in financial fraud detection.
Conclusion
The role of data science in financial fraud detection is transformative, enabling financial institutions to detect and prevent fraud more effectively than ever before. By utilizing techniques such as machine learning, anomaly detection, and predictive analytics, organizations can stay one step ahead of fraudsters and protect their assets. As financial systems become more complex and sophisticated, the role of data science will continue to grow, offering new opportunities for individuals to enter the field and contribute to the fight against financial fraud.