Highlights:
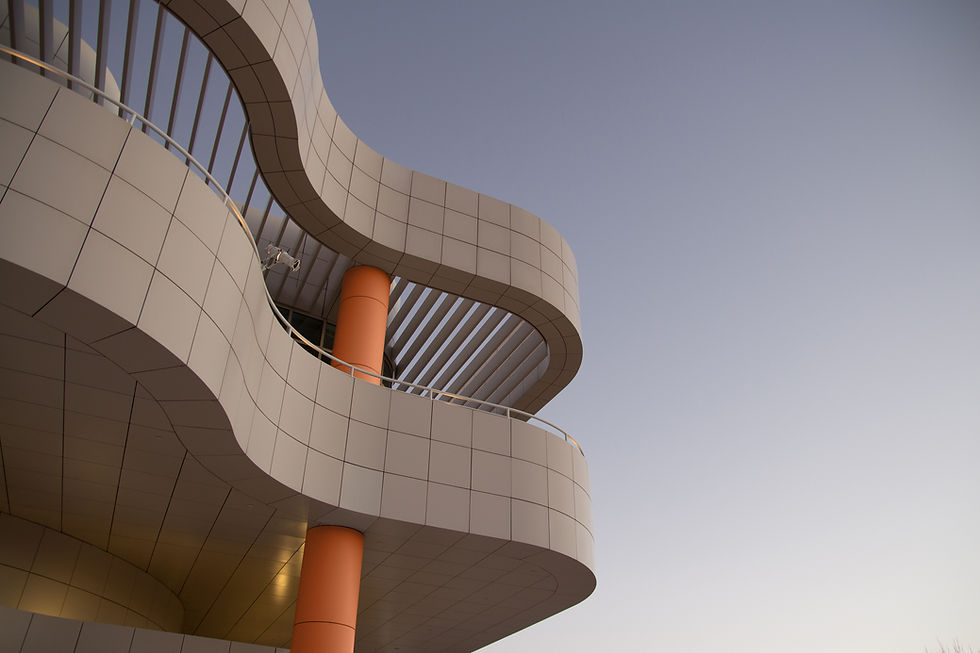
The Role of Deep Learning in Advancing Data Science
Sep 23, 2024
3 min read
0
1
0
In recent years, deep learning has emerged as a transformative technology within the field of data science. By leveraging complex neural networks, deep learning enables machines to learn from vast amounts of data, making it possible to uncover patterns and insights that were previously unattainable. This article explores how deep learning is advancing data science, highlighting its applications, benefits, and future potential.
Understanding Deep Learning
Deep learning is a subset of machine learning that mimics the workings of the human brain through artificial neural networks. These networks consist of layers of interconnected nodes, or neurons, that process data in a hierarchical manner. As data passes through these layers, the network learns to recognize intricate patterns, which can be applied to various tasks, including image recognition, natural language processing, and predictive analytics.
Applications of Deep Learning in Data Science
Image Recognition and Computer Vision: One of the most significant applications of deep learning is in image recognition and computer vision. Algorithms trained on large datasets can accurately identify objects, faces, and even emotions in images. For example, businesses use deep learning models to enhance security systems, automate quality control in manufacturing, and improve user experiences in social media platforms through personalized content.
Natural Language Processing (NLP): Deep learning has revolutionized natural language processing, allowing machines to understand and generate human language. Techniques such as recurrent neural networks (RNNs) and transformers enable applications like chatbots, sentiment analysis, and automated translation services. This advancement not only streamlines communication but also offers businesses insights into customer sentiment and preferences.
Predictive Analytics: In the realm of predictive analytics, deep learning models can analyze historical data to forecast future trends and behaviors. Businesses leverage these models for applications like customer segmentation, risk assessment, and sales forecasting. By understanding customer behavior patterns, organizations can make informed decisions that enhance profitability and customer satisfaction.
Healthcare and Medical Imaging: Deep learning plays a crucial role in advancing healthcare, particularly in medical imaging. Algorithms can analyze X-rays, MRIs, and CT scans with high accuracy, assisting radiologists in diagnosing conditions such as tumors or fractures. This technology not only speeds up the diagnostic process but also improves the accuracy of patient assessments, leading to better treatment outcomes.
Benefits of Deep Learning in Data Science
Enhanced Accuracy: Deep learning models often outperform traditional machine learning algorithms in accuracy, particularly with large datasets. Their ability to learn complex patterns makes them ideal for tasks like image and speech recognition.
Automation of Feature Engineering: Traditional data science approaches require manual feature extraction, which can be time-consuming and error-prone. Deep learning automates this process, enabling data scientists to focus on higher-level analysis and strategy.
Handling Unstructured Data: A significant advantage of deep learning is its capacity to process unstructured data, such as images, audio, and text. This capability expands the range of data types that can be analyzed, unlocking new insights for organizations.
Scalability: Deep learning models can scale effectively with increasing data volumes. As organizations gather more data, these models can adapt and improve without the need for extensive reconfiguration.
Challenges and Considerations
Despite its numerous benefits, deep learning is not without challenges. One significant concern is the need for large datasets to train effective models. In many cases, obtaining sufficient high-quality data can be difficult. Additionally, deep learning models can be computationally intensive, requiring significant resources for training and deployment.
Another challenge is the interpretability of deep learning models. Often referred to as "black boxes," these models can be difficult to interpret, making it challenging for data scientists to understand how decisions are made. This lack of transparency can hinder trust and adoption in critical applications, such as healthcare.
The Future of Deep Learning in Data Science
The future of deep learning in data science is promising. As technology continues to evolve, we can expect advancements in algorithm efficiency, model interpretability, and data accessibility. The integration of deep learning with other technologies, such as edge computing and IoT, will further enhance its capabilities, allowing for real-time data processing and analysis.
Moreover, as organizations increasingly recognize the value of data-driven decision-making, the demand for skilled data scientists proficient in deep learning will continue to rise. Education and training programs focusing on these skills will be essential in preparing the workforce for the future landscape of data science.
Conclusion
Deep learning is undeniably a game-changer in the field of data science, driving innovations across various industries. Its ability to analyze vast amounts of data, recognize intricate patterns, and make predictions positions it as a critical tool for organizations aiming to leverage data for strategic advantage. As we move forward, embracing deep learning will be vital for those looking to stay ahead in an increasingly data-driven world. For individuals seeking to enhance their skills, offline data science classes in Delhi, Noida, Lucknow, Meerut, Indore and more cities in India can provide valuable training in these cutting-edge technologies.