Highlights:
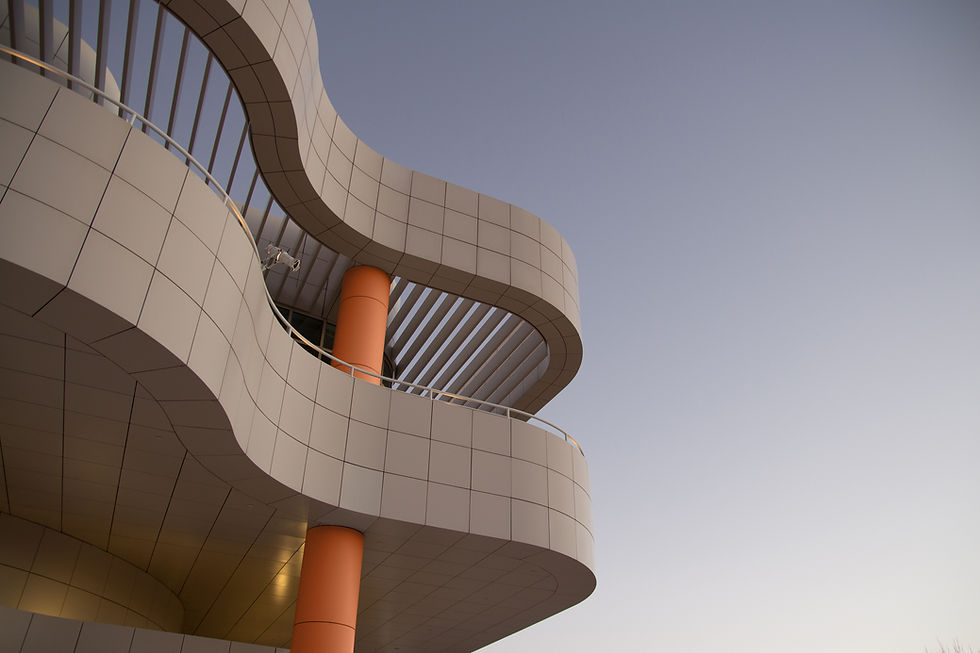
Top Programming Languages for Data Science Professionals
Nov 25, 2024
3 min read
0
2
0
In today’s data-driven world, data science professionals rely on programming languages to extract insights, analyze trends, and create models that drive decision-making. The choice of programming language plays a pivotal role in determining the efficiency, scalability, and success of data science projects. Here’s a look at the top programming languages for data science professionals and why they stand out.
1. Python: The All-Rounder
Python is often the first choice for data scientists due to its simplicity, versatility, and a vast ecosystem of libraries.
Why Python?
Libraries like NumPy, Pandas, and Matplotlib simplify data manipulation and visualization.
Machine learning frameworks such as TensorFlow, PyTorch, and Scikit-learn offer robust tools for model development.
Its ease of learning makes it ideal for beginners while offering advanced features for experts.
Use Cases: Data preprocessing, machine learning, and deep learning applications.
2. R: The Statistical Powerhouse
R is a language specifically designed for statistical computing and data visualization.
Why R?
Extensive libraries like ggplot2 and dplyr provide powerful tools for data analysis and visualization.
Excellent for statistical modeling and hypothesis testing.
Popular among academics and researchers for its statistical precision.
Use Cases: Statistical analysis, predictive modeling, and academic research.
3. SQL: The Data Query Expert
Structured Query Language (SQL) remains an essential tool for accessing and manipulating structured data in databases.
Why SQL?
Efficiently retrieves and manages large datasets.
A must-know for handling relational databases like MySQL, PostgreSQL, and Microsoft SQL Server.
Often used in conjunction with Python or R for data analysis workflows.
Use Cases: Data extraction, database management, and ETL (Extract, Transform, Load) processes.
4. Java: The Enterprise Choice
Java’s scalability and performance make it a preferred language for building big data solutions.
Why Java?
Integrates well with big data frameworks like Apache Hadoop and Apache Spark.
Highly reliable for enterprise-level applications and data pipelines.
Strong community support and extensive libraries for data science.
Use Cases: Big data applications, distributed systems, and large-scale data processing.
5. Julia: The New Contender
Julia is rapidly gaining popularity for its speed and focus on numerical computing.
Why Julia?
Offers performance close to C while maintaining high-level syntax like Python.
Well-suited for tasks involving large-scale numerical analysis.
Growing library support, including packages like DataFrames.jl and Flux for data manipulation and machine learning.
Use Cases: High-performance computing, large-scale simulations, and mathematical modeling.
6. Scala: The Big Data Specialist
Scala is known for its compatibility with Apache Spark, a leading big data processing framework.
Why Scala?
Allows seamless integration with Java libraries.
Provides functional programming features ideal for data pipelines.
Well-suited for big data processing and real-time analytics.
Use Cases: Big data processing, real-time analytics, and distributed computing.
7. MATLAB: The Engineering Favorite
MATLAB excels in mathematical and engineering computations.
Why MATLAB?
Simplifies working with complex mathematical problems.
Offers specialized toolkits for signal processing, control systems, and neural networks.
Used extensively in academia and industries like aerospace and automotive.
Use Cases: Engineering simulations, image processing, and algorithm development.
How to Choose the Right Language?
The right programming language depends on your goals, expertise, and the project requirements. Here are some tips:
Beginners: Start with Python for its simplicity and extensive support.
Statistical Focus: Opt for R if your work involves heavy statistical analysis.
Big Data: Choose Scala or Java for working with distributed systems.
Speed and Efficiency: Julia is a great option for high-performance tasks.
Conclusion
Mastering programming languages is crucial for data science professionals to stay competitive in a rapidly evolving field. While Python and R remain the go-to choices, other languages like Julia, SQL, and Scala have carved their niches in specialized domains.
By diversifying your skill set and adapting to project needs, you can position yourself as a versatile and in-demand data science professional. If you're ready to dive in, consider enrolling in a Data Science Training Institute in Pune, Mumbai, Chennai, Kolkata, Indore and more cities in India to gain hands-on experience and guidance. Start with the language that aligns best with your career goals and project aspirations!